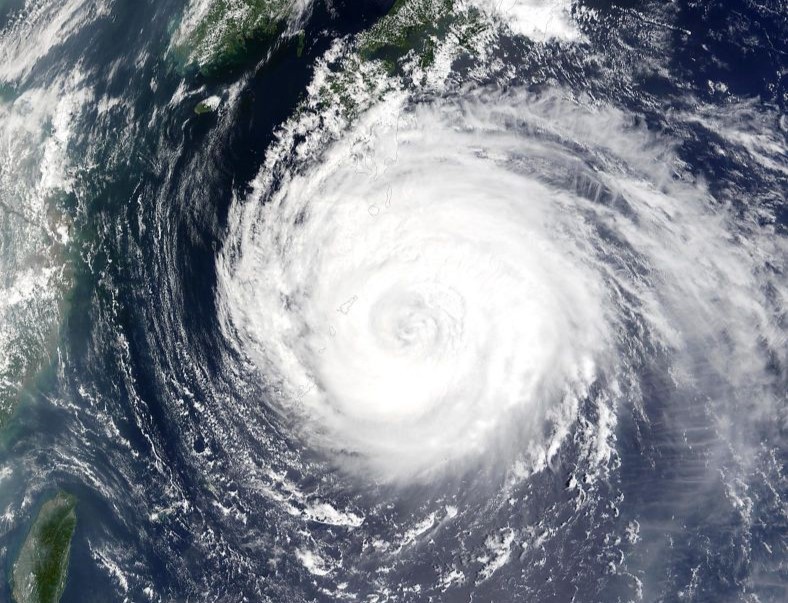
Scientists employed AI loaded with data from 627,400 tropical storms in the past 20 years in an effort to study the effects of ocean surface temperature on the ecosystem.
Impact of Ocean Surface Temperature on Ecosystems
Tropical cyclones, with their circular formation, thrive over warm tropical oceans, featuring low pressure, and high winds that reach above 39 mph for storms and 74 mph+ for hurricanes, in addition to heavy rain. These cyclones feed on warm waters, cooling the ocean's surface, while strong winds stir currents, bringing cooler water to the top.
AI predicts #seaSurfaceTemperature cooling during #tropicalCyclones @theAGU https://t.co/OCYFaAwkPk https://t.co/GSwsLNY8E8
— Phys.org (@physorg_com) September 26, 2023
This process reduces cyclone fuel, potentially slowing or halting them. Recent research utilizes AI technology to model cyclone effects on oceans, especially sea surface temperatures. This matters as temperature changes can impact ecosystems and marine life, making it vital for understanding and protecting ocean environments.
627,400 Tropical Cyclones in 20 Years
A team of scientists used a machine learning-driven random forest approach to analyze a 20-year dataset starting in 1998, focusing on predicting the spatiotemporal changes in sea surface temperatures within the northwest Pacific Ocean. The region, ranging from the equator to 30°N and spanning 100-160°E, is notorious for its high tropical cyclone activity.
Led by doctoral researcher Hongxing Cui from the Southern Marine Science and Engineering Guangdong Laboratory in China, the team utilized 12 key parameters related to tropical cyclones and pre-storm conditions to forecast cooling patterns in the Pacific Ocean basin.
The factors that have the most significant influence on subsequent ocean surface temperature variations include cyclone intensity, translation Speed, cyclone's size, and mixed layer depth.
Other factors include location, ocean surface height and temperature, temperature at 75 m depth, and changes in current speed.
The random forest model drew insights from historical data comprising 627,400 tropical cyclones spanning 20 years: 1998 to 2018. The AI uses data from cyclone activity in the three days leading up to an event and up to 14 days following its passage.
Cooling Ocean Surface and Formation of Tropical Cyclone
The study revealed a distinct cooling pattern in the days leading up to a tropical cyclone event, intensifying during the cyclone's passage and peaking one day after the event, resulting in a significant sea surface temperature drop of >1.3°C. If it reaches 2°C, category 3-5 hurricanes are observed.
This cooling effect extended over a larger ocean surface area, with the maximum cooling occurring about 50km to the right of the cyclone's direct path. Subsequently, from days two to four, the ocean underwent a relatively rapid warming process as it returned to normal conditions, followed by a slower recovery, reaching just 0.4°C above the local average by day 14.
In summary, the cooling effect on surface waters is more pronounced with stronger, larger, and slower-moving tropical cyclones in areas featuring a shallow mixed ocean layer. Storm intensity and speed have a localized impact, while cyclone size, pre-storm ocean mixed layer depth, and sea surface temperature influence cooling over a broader area.
Global Impacts
Comparing real data with random forest predictions, the researchers found a strong correlation, validating the machine learning model's potential for forecasting tropical cyclone impacts globally. This tool can aid in modeling effects in other ocean basins and assessing cyclone impacts on ocean ecosystems, including primary producers like photosynthesizing algae, crucial for marine food webs.
Related Article : Multi Vortex Tornado in Multiday Severe Weather Leaves Kansas, No Damages Reported
© 2025 NatureWorldNews.com All rights reserved. Do not reproduce without permission.