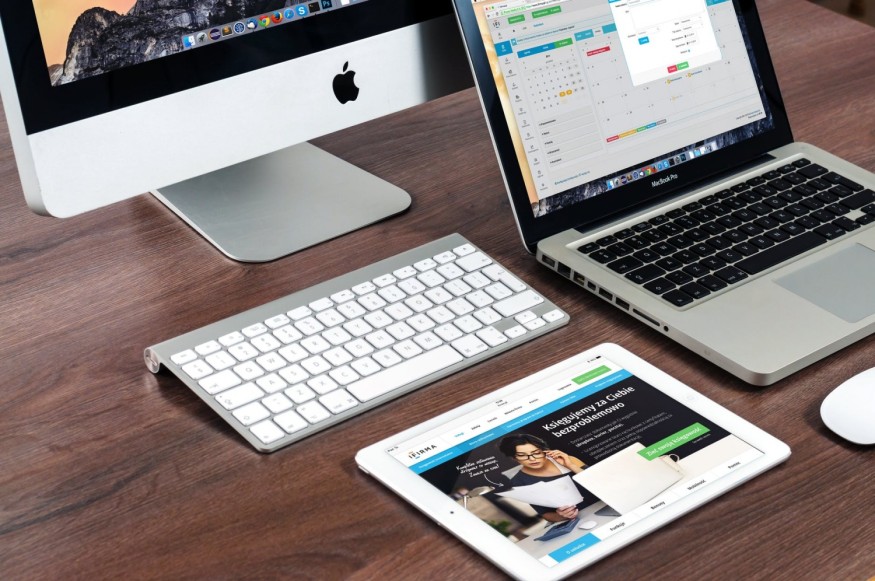
In 2017, Harry Glorikian and Malorye Allison Branca published MoneyBall Medicine: Thriving in the New Data-Driven Healthcare Market, showing us a picture of how data-driven reality will shape future health care delivery in the US.
The concept was patterned after the Moneyball concept put forward by Michael Lewis regarding the digitalization of professional football decision making. The authors have taken this thinking and applied it to health care, noting that when there are systems in place to collect and analyze data, they can be used to transform health care.
The motivation for writing the book came after observing how data technology transformed varied industries like football to the financial world. Glorikian notes that there is uncertainty in determining how AI, machine learning, and technology will impact health care. Resistance persists in health care when it comes to technology and data.
Data-driven practices may transform and improve the future of health care, Glorikian says. Transformation can begin with EHRs or electronic health records.
EHRs are not just a repository of laboratory data, radiology reports, and clinical notes anymore. Their enhancement can turn medicine into a proactive service; data can assist in identifying patients at risk for certain diseases and those due to certain diagnostic routines like cancer screenings.
Glorikian also notes how data analytics transforms medical practice with the advent of remote care; wearable, connected devices, and telemedicine.
He observed how there had been a massive shift to telemedicine and remote care during the COVID-19 pandemic. Such initiatives also help hospitals and health care systems be more efficient in the arena of value-based reimbursement.
Analytics and data also benefit from drug re-positioning and discovery. Traditionally, drug development has had an expensive price tag and took decades to conduct. Now, AI and computers can screen virtual molecules in libraries by the thousands, expediting the identification of molecules with the best chance for success.
Learning from COVID-19
Models are just as good as the quality of data. In the beginning, insufficient data plagued the coronavirus outbreak in policies on quarantine and social distancing. It did not help that the virulence of SARS-CoV-2 was not yet determined and that the health care infrastructure was not optimal.
Glorikian observed that it is daunting to determine the needed resources to deal with the outbreak and what policies to implement. Remarkable success is a speed of sequencing the virus' genome, which helped scientists better understand and predict its behavior. This positively impacts logistics and policymaking.
COVID-19 offers valuable lessons for dealing with future pandemics. Data help develop better models.
CEO and founder of Epic Judy Faulkner states that this pandemic will affect health care with the new data sharing and digital health care initiatives.
Challenges
Glorikian observes that it is only recently that patients have had access to their medical records online. Doctors are also still hesitant to depend on AI and analytic models. Standardizing data definitions is necessary for regulators like the CDC to access data rapidly. Faulkner notes that not doing so will prevent data from being aggregated and will render it useless.
Glorikian believes analytics and data will occupy a more central role in future health care management, and help us make better models from the data and lessons we learned from COVID-19, to handle future pandemics.
© 2025 NatureWorldNews.com All rights reserved. Do not reproduce without permission.