Lightning is one of nature's most devastating forces, as seen by the enormous California Lightning Complex fires in 2020, yet it's still difficult to forecast. According to a new study sponsored by the University of Washington, machine learning-computer algorithms that develop themselves without direct programming by humans-can be used to enhance lightning forecasts.
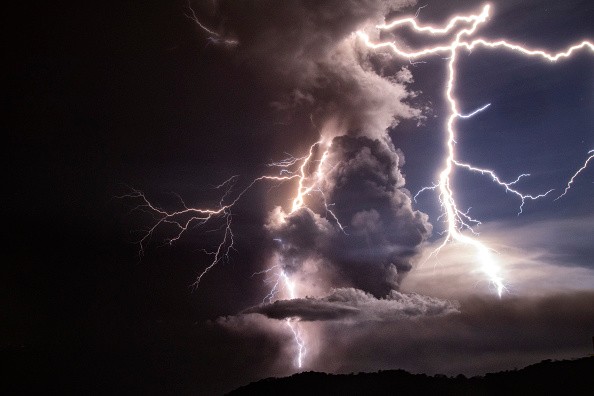
Improved Lightning Models
Better lightning predictions might aid in the prevention of wildfires, the improvement of lightning safety alerts, and the development of more accurate long-term climate models.
"The best subjects for machine learning are things we don't fully understand," said Daehyun Kim, a UW associate professor of atmospheric sciences. "And what is something in the atmospheric sciences field that remains poorly understood?" "To our knowledge, this is the first time that machine learning methods have been demonstrated to work for lightning."
Weather Forecasts

Weather forecasts are combined with a machine learning equation based on assessments of previous lightning incidents in the new approach. The hybrid method presented at the American Geophysical Union's autumn conference on Dec. 13 can anticipate lightning two days sooner than the leading existing strategy over the southeastern United States.
"This shows that utilizing machine learning approaches, predictions of severe weather systems like thunderstorms may be improved," said Wei-Yi Cheng, who completed the research for his Ph.D. in atmospheric sciences at the University of Washington. "It stimulates the use of machine learning algorithms for other forms of extreme weather forecasts, such as tornadoes or hailstorms," says the study.
Related Article: Study Warns US Residents to Prepare for Stronger Hurricanes in Next 100 Years
Using Lightning Data
Researchers used lightning data from 2010 to 2016 to train the algorithm, which allowed it to uncover correlations between meteorological factors and lightning strikes. They then put the strategy to the test on weather from 2017 to 2019, comparing the AI-assisted method to an existing physics-based method and evaluating both using actual lightning sightings.
In places with a lot of lightning, like the southeastern United States, the new method could forecast lightning with the same accuracy as the leading technique about two days earlier. Because the method was tested across the entire United States, it didn't perform as well in areas where lightning is less common.
Innovative Method
A recently developed strategy for forecasting lightning based on precipitation and the ascent speed of storm clouds was utilized for comparison. This technique has predicted greater lightning and a sustained rise in lightning over the Arctic with climate change.
"The conventional technique just combines two variables," Kim explained. "This is a human-created notion, and it's straightforward, but it's not the ideal way to use these two variables to forecast lightning."
The machine learning model was developed using data from the World Wide Lightning Location Network, a UW-based collaboration tracking worldwide lightning since 2008.
"One of the key requirements for a machine-learning algorithm to achieve some important things is that it has a lot of data," Kim added. "This would not have been conceivable five years ago since we didn't have enough data, even from WWLLN."
Lightning Monitoring Sensors
Commercial networks of lightning-monitoring sensors currently exist in the United States, and newer geostationary satellites can continually monitor one area from space, providing the accurate lightning data needed for greater machine learning.
"The number and quality of the data are the most important variables," Cheng explained. "WWLLLN can give us both." "An accurate and trustworthy lightning observation dataset will become increasingly crucial as machine learning techniques improve."
The researchers seek to enhance their system using new data sources, more weather factors, and more advanced algorithms. They'd like to improve forecasts for specific scenarios like dry lightning or lightning that occurs without rain because they are particularly deadly for wildfires.
Creating Long Term Forecasts
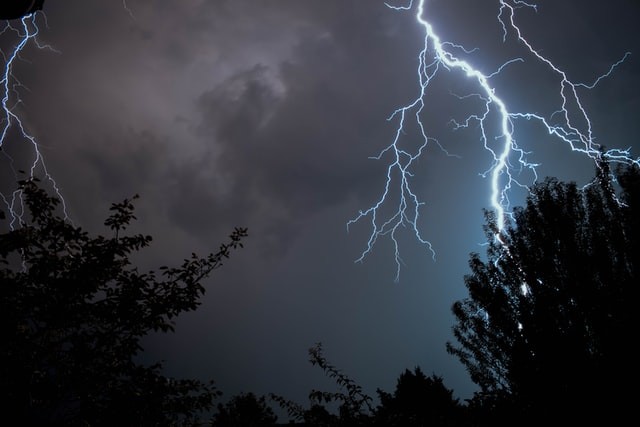
The researchers believe their strategy might be extended to longer-term forecasts as well. Longer-term patterns are essential because lightning impacts air chemistry. Therefore forecasting lightning improves climate models.
"Some people are still cautious about the application of machine learning algorithms in atmospheric sciences, as in other disciplines-because as scientists, we don't trust something we don't understand," Kim added. "I was a skeptic at first, but after viewing the findings of this and other studies, I'm sold."
Also Read: Texas Freeze Survivor Uses Tiktok to Help Others Prepare for Intense Winter
For more news about the environment , don't forget to follow Nature World News!
© 2024 NatureWorldNews.com All rights reserved. Do not reproduce without permission.